
Spatial Analysis of the Gender Pay Gap in China: Asymmetries as a Consequence of Development
Abstract
Using data from the Chinese Household Income Project and the China Family Panel Studies, this study shows the geographical characteristics of the gender pay gap in China and further underlines the relationship between the increasing gap observed between men’s and women’s wages and patterns of regional socioeconomic development. It contributes to the field by using a socioeconomic clustering of regions, which improves on the classical and simple geographical approach and analyzes the impact on the gender pay gap in each cluster. The spatiotemporal analysis from 1995 to 2018 shows that China's gender pay gap depends on development levels. It increases with province size, in terms of GDP and population, and the higher the foreign exchange earnings from international tourism, the smaller the gap.
Keywords:
China, cluster analysis, factor models, gender pay gap, regional analysisIntroduction
Although equality between women and men was enshrined as a core tenet of the UN Charter in 1945, 75 years later, women and girls live in a world of widespread gender inequality (UN, 2020). According to the latest information from the United Nations, countries are making progress on gender equality in line with the Millennium Development Goals (including gender equality in primary education), but women and girls worldwide still suffer from discrimination and violence.
In 2015, the United Nations approved the 2030 Agenda for Sustainable Development (UN, 2015), an opportunity for countries and their societies to embark on a new path toward improving the lives of all people, with no exceptions. The agenda has 17 sustainable development goals, including gender equality and empowerment of all women and girls. The agenda focuses on eliminating all forms of discrimination against women and girls worldwide, with reforms in accordance with national laws that will give women equal access to economic resources, the right to ownership and control of land and other forms of property, and access to financial services.
In this context, and also highlighting the fact that the UN 2030 Agenda has now entered the critical period of its remaining ten years, we find that, according to the Global Gender Gap Index 2020 (World Economic Forum, 2020), which captures the magnitude of gender-based disparities and tracks their progress over time, allowing country-by-country comparison, China ranks 106th out of 153 countries in the world, far behind Iceland and the Scandinavian countries, which occupy the top positions. Moreover, China has fallen three places from its previous ranking in 2018, indicating a decline, or at least a lesser advance, than other countries. China’s ranking is because, among other reasons, many women still face major barriers to economic and political advancement. For example, women’s representation on corporate boards or as top business leaders is 9.7%, while in France or Iceland this percentage is above 43%, with an OECD average of 22.3%. Moreover, in China, women receive approximately three-quarters of what men are paid in terms of base pay, performance pay, and total pay (Xiu & Gunderson, 2013) and China's urban labor market seems to increasingly disincentivize women's long-term labor force attachments (Hare, 2019). In addition, Evans (2021) highlights an apparent paradox between women’s increasing access to education and employment and their abiding attachment to ideas and practices associated with their roles as wives, mothers, and daughters-in-law.
One of the most relevant indexes in terms of gender inequality in the labor market, the gender pay gap (the difference between the average earnings of men and women), is attracting increasing attention in China. Following Gustafsson and Li (2000), the average gender earnings gap appeared in 1988–1995 to be relatively small by international standards, though increasing from 15.6% to 17.5%. At the same time, in 1995, in the United States it was 24.6%, in the European Union 17%, and the United Kingdom 28.2%. Later, Chi and Li (2014) stated that it increased from 18% in the late 1980s and early 1990s to 25% in 2009, and the size of the gap may still be an underestimate as a result of not accounting for low-wage women who have dropped out of the labor force. The most recent data, obtained from the China Family Panel Studies (CFPS) for the year 2018, show that the gender pay gap in China's annual earnings has reached 26.5%, compared to 18.9% in the United States, 16% in the European Union, and 16.3% in the UK (OECD, 2020; CFPS, 2018). Note that the gender pay gap has increased by 11 percentage points in China over the past 30 years.
History tells us that traditionally, China has long emphasized the subordinate roles of females in society. However, after the founding of the People's Republic of China in 1949, China implemented a planned economic system and national wage scales based on socialist egalitarian principles, whereby wage disparities due to gender and human capital were abolished (Xiu & Gunderson, 2013). The Communist Party promoted and promulgated the rhetoric and ideology of gender equality (Hughes & Maurer-Fazio, 2002).
Beginning in 1978, China carried out a process of economic opening, from a planned economy to a market economy. The process of market liberalization was not straightforward, and even today the market transition process in China is far from complete (Naughton, 2007). The gradual opening up of the market through reforms has had a significant impact on national and international investment in the Chinese national economy. The process of labor movement toward private enterprise has also brought about increased inequality in the distribution of wages among workers in urban China (Whalley & Xing 2016).
It seems clear that the gender pay gap has also suffered as a result of this movement toward a free market economy. Ng (2007) finds that both market competition and the effects of wage decentralization play a role in shaping gender earnings differentials. Several authors state that because of China’s abundant labor force and ingrained Confucian patriarchal social norms, the Chinese government’s relaxation of rules governing the economy may also encourage a resurgence of workplace gender discrimination (Chen, Ge, Lai, & Wan, 2013; Hughes & Maurer-Fazio, 2002; Knight & Song, 2003; Ng, 2004). Xiu and Gunderson (2013) state that the gender wage gap is larger in more competitive and smaller in less competitive sectors, including the state-owned and collective sectors. Chen et al. (2013) find that a large gender wage gap exists for foreign and exporting firms, but this mainly reflects differences in gender productivity, as female workers are more likely to be assigned to low-tech and low-training jobs.
Not all Chinese provinces have experienced the same level of development, nor has that development occurred at the same speed. From a spatial point of view, since economic reform in China has resulted in uneven economic growth and development when comparing the coastal eastern provinces and the provinces of the central and western regions, it is to be expected that the consequences for the wage gap would also be different in each region. Regional factors cannot be neglected in any earnings differential study of a large transitional economy such as China’s (Ng, 2004). What, therefore, are the geographical characteristics of the gender pay gap in China? Is the increasing gap related to changes in China’s policy of economic reform and openness? Gustafsson and Li (2000), who examined ten provinces, stated that differences in earnings levels across provinces were quite significant compared to differences in earnings along other dimensions such as age or education. Studying the gender pay gap between 1988 and 1995, when China experienced rapid industrialization, urbanization, and transformation toward a market economy, they found that in segments of urban China where market forces gained in influence, earning differentials between women and men increased more rapidly than in other areas. Ng (2007) also found that, in regions of China that experienced rapid development, more flexible pay ranges had an even greater discriminatory effect.
While three regions have traditionally been considered in research studies—the eastern, central, and western regions—a multivariate data analysis approach can achieve a classification that is more in line with the socioeconomic development of each group. The present study attempts to improve on the work reported in the literature related to the Chinese economy by identifying the relationship between socioeconomic regional development and the gender wage gap. The main novelty of this study is that it contributes to the research field by examining regions as socioeconomic clusters and analyzing the impact on the gender pay gap in this spatial aggregation.
The rest of the study is structured as follows. Section 2 describes the data and methodology applied, and in Section 3, clusters of Chinese provinces are created according to their socioeconomic characteristics using a multivariate approach. A factor analysis was conducted to identify the most relevant latent factors. Section 4 presents the results of the data regarding the gender pay gap in Chinese provinces and clusters, and Section 5 includes the final discussion and conclusions.
Data and Methodology
Database for Computing the Gender Pay Gap
The analysis of the gender pay gap in China is mainly based on data from the Chinese Household Income Project (CHIP, 2020). These surveys were compiled by the Income Distribution Research Institute of Beijing Normal University, a scientific research institution whose main interests are income distribution, the labor economy, and economic development. The CHIP database was created using data from household surveys completed in 1988, 1995, 2002, 2007, and 2013, and it is considered the most authoritative source of information in the field of income distribution and research into the labor market in China. In this article, data sets from 1995, 2002, and 2013 are used1 (see Table SM.1 in the supplementary material).
The sample eliminates observations of the retired and unemployed, school students, disabled people, and others who cannot work for various reasons. In general, those included in the sample will have completed paid work for more than one consecutive month by the end of the year, including casual work.
With the aim of adding more updated information to the study, we also considered data from the 2018 China Family Panel Studies (CFPS, 2020), a project authorized by Peking University, and the National Natural Science Foundation of China. The CFPS sample, which began in 2010 and is conducted on a biannual basis, covers 26 provinces, municipalities, and autonomous regions. The available database contains 11,513 data items. In some provinces, such as Fujian, sample sizes can be small; therefore, the results, while valid in general and not detracting from the validity of the analysis, should be interpreted with caution.
The data on annual earnings provided directly by CHIP and CFPS include total fixed wages, variable wages, overtime pay, allowances and bonuses, end-of-year bonuses, rewards in kind (converted into cash), second occupations, part-time or temporary income, and other income gained through working.
Data for Socioeconomic Classification
Socioeconomic data were obtained from the National Bureau of Statistics of China (NBS). The data contain social, economic, cultural, and other information about China’s 31 provinces. The analysis did not include Hong Kong, Macao, or Taiwan. Table SM.2 shows the variables considered to characterize each region’s socioeconomic level.
Gender Pay Gap Methodology
Using annual earnings data, a first approach to the official concept of the gender pay gap can be carried out using the following formula:
(1) |
This measure reflects the difference in annual earnings by gender and informs us about the ability of women to support themselves and the implications for their retirement pensions of earning less money per year throughout their tax period as compared to men.
However, the calculation of the gender pay gap in annual earnings does not take into account individual differences, such as the number of hours worked. Thus, the gender pay gap should represent the difference between the hourly earnings of men and women. The European Commission defines the gender pay gap as the difference between the average gross hourly earnings of men and women, expressed as a percentage of men’s average gross hourly earnings (European Commission, 2020). Therefore, we also analyze the gender pay gap for hourly earnings. The formula used is as follows:
(2) |
Factor Analysis and Cluster Methodology
To carry out a socioeconomic characterization of China’s provinces, a large number of variables and observations of these variables is required. This therefore requires the use of multivariate analytical techniques. Specifically, considering the objectives set, the multivariate techniques used are factor analysis and cluster analysis (Hair, Anderson, Tatham, & Black, 1998; Harman, 1976; Manly, 2004). Factor analysis is intended to determine unobserved causes or factors that determine the differences between provinces, while cluster analysis will attempt to divide China into groups of homogeneous provinces.
In particular, to proceed with the extraction of the factors, the principal component method was used to carry out the correlation analysis from standardized variables, with the requirement that the underlying factors be independent. Likewise, and in order to solve the usual problem of interpreting the meaning of the factors, we have used the Varimax method of rotation of the axes, which seeks to maximize/minimize the correlation of each variable with each factor—details of these techniques and their alternatives can be found in Kaiser (1958), McDonald and Burr (1967), Gorsuch (1983, 1990), Jollife (1986), Stevens (2001), and Romesburg (2004), to mention just a few.
Having reduced the number of variables, cluster analysis seeks to classify a set of individual items (provinces of China) into groups, unknown a priori, including the most internally homogeneous and heterogeneous among them. One of the key questions in the application of this technique lies in the choice of similarity measures. Among the options, the Mahalanobis distance was chosen, which maintains the advantages of the Euclidean distance, discounting the effect of over-accounting for discrepancies introduced by the existing correlation between the variables. The categorization of the provinces was carried out employing a hierarchical clustering procedure using Ward's method. This seeks to maintain the maximum possible homogeneity within each group with minimal distortion with respect to the original data to measure the distance between groups—details on the advantages and disadvantages associated with this approach and the alternatives can be consulted in Anderberg and Blashfield (1973) and Everitt (1993), among others.
Socioeconomic Clusters of Chinese Provinces
Economic development in China and its transition to a free-market economy have not occurred at the same rate nationwide, which has resulted in different socioeconomic levels in different provinces. China's imbalance in regional economic development has attracted widespread attention. Song (2013) states that market openness provides different development opportunities for different regions; high levels of openness lead to high and rapid economic development, while in other areas, development lags behind. In 2000, China’s government presented the Great Western Development Strategy, specially adapted to develop the western regions of China, including Gansu, Guizhou, Qinghai, Shaanxi, Sichuan, Yunnan, Guangxi, Inner Mongolia, Ningxia, Tibet, Xinjiang, and Chongqing. A similar plan was designed in 2004 to accelerate the development of its central regions through the Rise of Central China Plan, affecting Shanxi, Henan, Anhui, Hubei, Hunan, and Jiangxi, followed in 2010 by the Northeast Area Revitalization Plan. There are also several provinces and municipalities along the Yangtze River, China's longest river, which have been designated as having important roles in promoting innovative green development strategies for the world's second-largest economy. The Yangtze River Economic Belt covers about a fifth of China’s territory, has a population of 600 million people, and generates 40% of the country's GDP. It is made up of nine provinces (Sichuan, Guizhou, Yunnan, Hubei, Hunan, Jiangxi, Anhui, Jiangsu, and Zhejiang) and two municipalities (Chongqing and Shanghai). Despite these plans, or while still waiting for their effects to be visible, Ju (2020), through a decomposition of the Theil index, states that the imbalance in China's economic development comes mainly from regional differences. According to Jianhua and Jun (2019), from 1990 to 2016 the gap between the central and western regions and the eastern region has widened in terms of GDP per capita.
The aim of this section is to group the provinces in such a way that those belonging to the same group are as similar as possible to each other in terms of their socioeconomic characteristics and are as different as possible from the provinces of the other groups. To obtain this clustering of the provinces based on their socioeconomic characteristics, the information related to the 14 variables reported in Table SM.3 was considered. Figure SM.1 shows their box plots.
Based on this information, we identified the factors that characterize socioeconomic structure using the factor analysis technique (as in Larraz and Pavia, 2010). These factors summarize the information available for the large number of variables considered. Prior to the factor analysis, it was detected that the CPI variable does not provide enough information for the analysis; it does not have enough information in common with the rest of the variables to be convenient for inclusion in the analysis. Therefore, given a measure of sampling adequacy (MSA) for this individual variable of 0.22 (<0.5), it was eliminated from the subsequent analysis. Of the 13 remaining variables, with an overall Kaiser-Meyer-Olkin (KMO) factor adequacy of 0.54, the first five factors were selected, representing an accumulated 83.9% of the total variance and were related to the five eigenvalues greater than 1.
These five factors have been given the following labels due to their correlations with the original variables, which can be seen in Table SM.3.
Factor 1: Wealth Factor. This factor is strongly positively correlated with GDP per capita and foreign exchange earnings from international tourism and life expectancy. Provinces with high score in this factor have good living standards in economic terms when compared with the rest of the country, as well as higher life expectancy.
Factor 2: Size Factor. This is strongly positively correlated with global GDP and the population of the province. The higher the score in this factor, the bigger the province in terms of population and GDP.
Factor 3: Energy consumption and transport factors. This factor is positively correlated with electricity and gas consumption and with the number of vehicles per capita. Provinces with high score in this factor are those that consume more energy, perhaps because they correspond to regions where heavy industry is prevalent and with a large proportion of urban areas.
Factor 4: Society Factor. The name stems from the positive correlation of this factor with the number of libraries and hospitals per capita. This is inversely correlated with the death rate.
Factor 5: Unemployment Factor. Provinces with high score in this factor suffer from a high unemployment rate and, as a consequence, a low birth rate. Therefore, it is a negative factor from a socioeconomic perspective.
Using these five factors, which provide a characterization of the socioeconomic level of China’s provinces, a cluster analysis was developed to create groups with similar characteristics. Following the approach detailed in the methodology section, we obtained nine groups, which are reported in Table SM.4 and the corresponding dendrogram is shown in Figure 1. Taking into account the mean scores for each group in each factor, reported in Table SM.5, we determined why the provinces were included in each cluster. Consequently, we deduced the characteristics that the provinces of each group have in common, and which differentiate them from the other provinces.
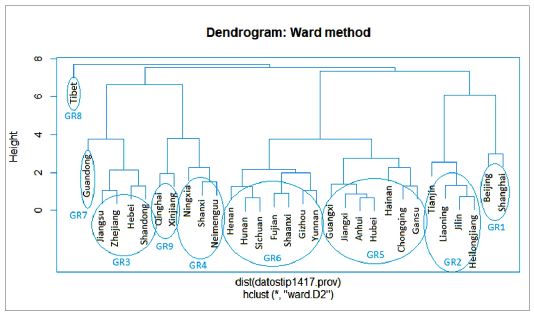
Dendrogram showing the provinces that are at a shorter distance and, therefore, the groups resulting from the clustering technique.Note. Author’s elaboration.
There are two groups containing only one province: Guangdong in Cluster 7 and Tibet in Cluster 8, indicating these two provinces are significantly different from the rest of the country. Guangdong is the biggest province, not in terms of area but in terms of GDP and population, with 113,460,000 inhabitants in 2018, far ahead of Shandong, the next largest by population. It has one of the lowest unemployment rates in China at 2.4%, compared to the highest level registered in Heilongjiang (4%), and a high birth rate (12.79‰, with the highest being Tibet’s 15.22‰). On the other hand, Tibet stands out for having the largest number of libraries and hospitals per million inhabitants, as well as having one of the lowest death rates (4.58‰, compared to a national average of 7.08‰).
Cluster 1 is made up of Beijing and Shanghai, the provinces with the highest GDP per capita, followed by Jiangsu and Zhejiang (which belong to Cluster 3). They also present a high level of foreign exchange earnings from international tourism, followed by Guangdong (Cluster 7), and the highest life expectancy (above 80 years).
Another cluster with only two provinces is Cluster 9, which contains Qinghai and Xinjiang. They are provinces with low unemployment rates (3% and 2.4%, respectively, this last being the same as Guangdong). Qinghai has one of the highest birth rates. They are relatively small in terms of population, and produce little compared to the other provinces. Their GDP per capita is also low.
Provinces belonging to Cluster 4 are characterized by their high per capita energy consumption. Neimenggu (Inner Mongolia), which has an abundance of natural resources, along with Ningxia and Shanxi, are inland provinces located in northern China. Ningxia and Neimenggu have the highest electricity consumption per capita and are the most important energy supply zones in China, containing large quantities of coal, wind, and solar resources. This has led to the setting up of many large mining and smelting companies in this area, industries that consume large amounts of electricity. All three also have a relatively high gas consumption per capita. They are below average in wealth and size, and above average in the “society” factor, with an above-average unemployment rate.
The second cluster, Cluster 2, formed by Heilongjiang, Jilin, Liaoning, and Tianjin, all provinces in eastern and northern China, is characterized by being an area with a relatively high unemployment rate, over 3.5%. This reality combines with the lowest birth rate in China (around 6‰) and a relatively low GDP per capita, particularly in Heilongjiang (33,977 yuan/inhab, the second with lowest GDP per capita after Gansu).
Cluster 3 is composed of Hebei, Jiangsu, Shandong, and Zhejiang, all coastal provinces in eastern China. They are relatively large areas in terms of population (all have more than 57 million inhabitants) and global GDP. Jiangsu, Shandong, and Zhejiang are the provinces with the highest GDP after Guangdong. They have high rates of per capita electricity consumption and vehicle ownership, with Zhejiang the highest at 23 vehicles per 100 inhabitants.
Finally, Clusters 5 and 6 are larger groups, made up of seven provinces each. All factors for the provinces of Cluster 5 are below average. It comprises Anhui, Chongqing, Gansu, Guangxi, Hainan, Hubei, and Jiangxi, all of which are in inland China. They have relatively low electricity consumption, a below-average number of hospitals, the lowest number of vehicles per 100 inhabitants (less than 12 units), and great variability in gas consumption, number of libraries, foreign investment, and life expectancy. All have a birth rate above 11‰ and a medium high death rate (> 5.9‰). As a consequence, they have a relatively low unemployment rate, with all the provinces under 3%, except Jiangxi (3.4%).
Cluster 6 presents provinces with average values around the mean: a slightly below-average score in wealth factor, energy consumption, and society, and a slightly above-average score in size and unemployment. It comprises Fujian, Guizhou, Henan, Hunan, Sichuan, Shaanxi, and Yunnan. The score of these provinces generally presents great variability. In terms of GDP per capita in particular, the range is from Guizhou (42,767 yuan/inhab) to Fujian (98,542 yuan/inhab). In GDP and population, they are relatively large provinces (the smallest is Guizhou with 36 million inhabitants); unemployment is relatively high; energy consumption, vehicle ownership, and life expectancy are below the average in almost all of them but with great variability; and birth rates and death rates are medium-high.
Results: Spatial Analysis of the Gender Pay Gap
Gender Pay Gap Evolution from 1995 to 2013
The Yangtze River, the largest river in China and the river with the highest volume of cargo in the world, holds an important strategic position in the general scheme of regional development. From 1995 to 2013, the gender pay gap in the Yangtze River Economic Zone (Jiangsu, Anhui, Hubei, Chongqing, and Sichuan) increased by 10–17 percentage points. With the rapid economic development in these provinces, the gender pay gap between men and women has gradually widened. In contrast, the gap in the northern provinces (Gansu, Shanxi, Henan, Beijing, Liaoning) has remained practically the same or has even fallen, such as in the provinces of Gansu, Henan, and Liaoning (see Figure 3).
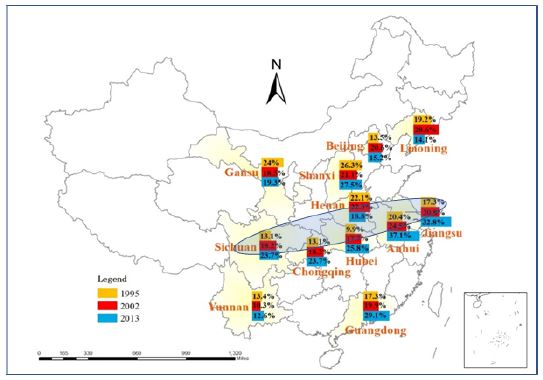
Gender pay gap related to average annual earnings by province (1995, 2002, 2013).Source. Compiled by the authors with data from CHIP 1995, CHIP 2002, and CHIP 2013.
Among the southern provinces, the gender pay gap in Guangdong also widened (12 percentage points) due to the effect of national policies and the rapid development experienced by the province from 1995 to 2013. The gender pay gap in Yunnan Province is relatively low compared to other provinces, at 13.4%, 10.3%, and 12.6%, respectively, for the three years of the survey.
The gender pay gap in hourly earnings shows that the situation in the northern and southern provinces is completely different (see Figure 4). The gap in most of the northern provinces (Gansu, Shanxi, Henan, and Liaoning) decreased between 1995 and 2013. The most obvious was Liaoning, where the gender pay gap in hourly earnings fell from 18.7% to 12.1%. However, between 1995 and 2013, the gap increased in the southern provinces (Sichuan, Chongqing, Hubei, Anhui, Jiangsu, Yunnan, and Guangdong). In the Yangtze River Economic Zone, the gender pay gap increased significantly, between 9 and 12 percentage points. In particular, in Hubei Province, it increased from 10% to 25.5% (15.5 percentage points between 1995 and 2013.
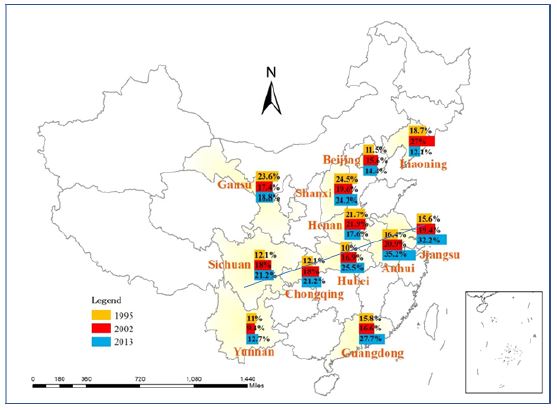
Gender pay gap related to average hourly earnings by province (1995, 2002, 2013).Source. Compiled by the authors with data from CHIP 1995, CHIP 2002, and CHIP 2013.
The map shows several provinces with values above the national average of 26.5%. The coastal province of Fujian has the largest gender pay gap at 55.76%, which means that the annual earnings of women on average in the province represent only 44.24% of that of men. This anomalous result could be explained by the province’s relatively small sample size (n = 145). The distribution shows that there are many men with higher salaries than women, not just one rich man. However, these results should be interpreted with caution. In Jiangsu, Jiangxi, Anhui, Hubei, and Sichuan, belonging to the Yangtze River Economic Zone, the gender pay gap reaches 30.55%, 35.77%, 42.01%, 42.48%, and 32.78%, respectively, very high in comparison with the less developed provinces (from a socioeconomic point of view) and significantly higher than the average gender pay gap in China.
The regions with smaller gender pay gaps are mostly concentrated in inland provinces, such as Gansu (25.17%) in the north and Guizhou (21.98%), Hunan (20.32%), Chongqing (22.22%), Guangxi (19.4%), and Yunnan (26.35%) in the south, all lower than the national average of 26.5%. They also have relatively low annual earnings. Finally, the smallest gender pay gap can be seen in Tianjin (11.24%), a major port city in northeast China with relatively high wages for women and men, and in Shanghai (15.44%). Finally, Beijing, the province with highest annual salaries, shows a gender pay gap of 23.98%, lower than the national average.
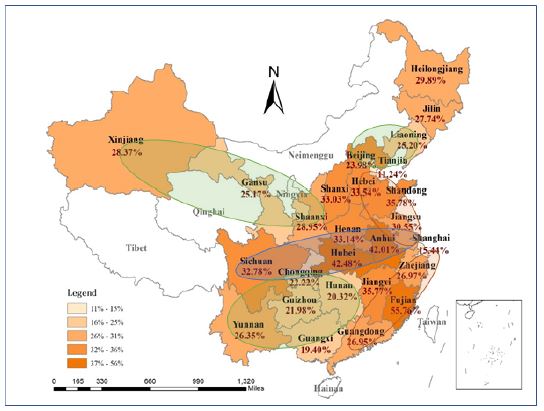
Gender pay gap related to annual earnings by province in 2018.Source. compiled by the authors with data taken from CFPS 2018.
Regarding the gender pay gap for hourly earnings, Beijing and Shanghai, which have the highest hourly wages, have lower gender pay gaps for hourly earnings, with 17.41% for Beijing and 11.96% for Shanghai, far below the average level in China (see Figure 6). The study also found that the gender pay gap for hourly earnings in eastern and coastal areas is higher than that in inland areas. For example, Hebei, Shandong, Jiangsu, Fujian, and other provinces have a higher gender pay gap in wages per hour. The gap for hourly earnings in the Yangtze River Economic Zone is also relatively high, such as in Sichuan, Hubei, Anhui, and Jiangsu provinces (except Shanghai). On the other hand, the gender pay gap for hourly earnings of the inland provinces is relatively low (see data from Xinjiang, Gansu, Shaanxi, Yunnan, Guizhou, Hunan, and other provinces). At the same time, their hourly wages are also relatively low.
The Gender Pay Gap in the Socioeconomic Clusters
Analyzing the gender pay gap for the provinces of each cluster, we observe that clusters 1 and 2 are made up of provinces with low gender pay gaps, compared with the rest of the provinces in China (see Figures 7 and 8). The provinces in clusters 3 and 4, however, present medium-high gender differences in wages, while clusters 5 and 6 present great variability, containing provinces with a high gender pay gap and others with a low one. Note that these two groups are made up of provinces with great variability in some variables, which can account for this difference in the behavior of their gender pay gaps. Guangdong, which constitutes Cluster 7, is average, while Cluster 9 has a low gender pay gap for hourly wages.
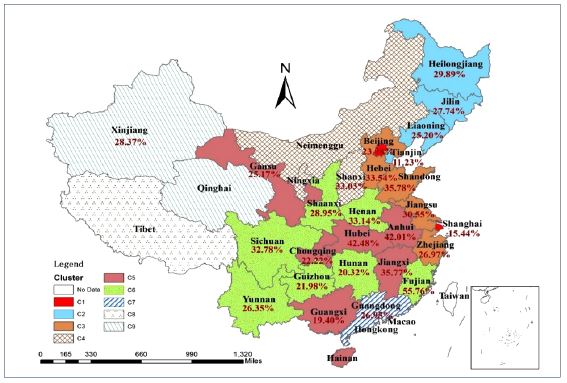
Gender pay gap related to annual earnings and socioeconomic clusters in 2018.Source. compiled by the authors with data taken from CFPS 2018.
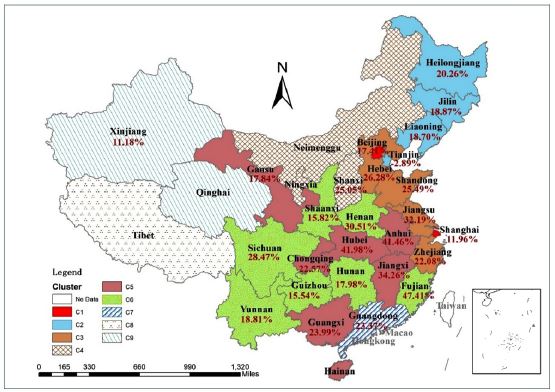
Gender pay gap related to hourly earnings and socioeconomic clusters in 2018.Source. compiled by the authors with data taken from CFPS 2018.
This behavior of the gender pay gap can be associated with the factors identified in Section 3 through the linear correlation coefficient (see Table SM.6). The highest correlation coefficient, between the size factor and the gender pay gap measured on annual wages, is r = 0.476. This indicates that, at most, the linear correlation was moderate. This implies that the gender pay gap depends to a moderate extent on the factors and variables identified, and does not strongly depend on them. Bearing in mind that this correlation is medium, in general, the gap increases with the size of the province in terms of GDP and population (r = 0.45). All provinces with more than 50 million inhabitants or with production above 3,800,000 million yuan have a gender pay gap above the average. The gap is not related to the GDP per capita of the province, but in general, the higher the foreign exchange earnings from international tourism, the lower the gender pay gap, which implies that in those provinces with high score in the wealth factor, the gap is low, especially in terms of hourly wages. This is true for Shanghai, Beijing, Yunnan, Shaanxi, and Tianjin. The exception, where the correlation is only moderate, is Fujian.
In addition, the gender pay gap has a positive correlation with the birth rate (r = 0.47), which implies a negative correlation with the unemployment factor, but there are several exceptions. For example, provinces with birth rates below the first quartile present a low gender pay gap, as Shanghai, Beijing, Tianjin, Liaoning and Jilin. The correlation between the gender pay gap and energy consumption is weak, but gas consumption is negative (r = -0.46). In general, provinces with high gas consumption present a medium-low gender pay gap (Beijing, Tianjin, Xinjiang, and Shanghai). In contrast, Jiangxi, Anhui, and Hubei have a gender pay gap above the third quartile and low gas consumption per capita. Finally, in the case of the society factor, the negative correlation is caused by the number of hospitals (r = -0.47). Xinjiang, Guizhou, Liaoning, Shaanxi, Beijing, and Jilin have more than 28 hospitals per million inhabitants. Note that the linear correlation coefficient does not imply causality.
Fujian deserves special attention because it had the highest gender pay gap in China in 2018, although we cannot know its trend because it was not included in the survey until 2018. It belongs to Cluster 6. We have already pointed out that Fujian is an exception, and this large gender pay gap can be related to its rapid GDP development in recent years. From 2012 to 2018, Fujian's GDP growth rate has been above 8.1%, much higher than China’s average national growth rate. It has a high GDP (1.0823 billion USD) and was able to grow at a rate of 8.3% in 2018. Its proximity to Taiwan may be a factor in this. It has low gas consumption and a fewer number of hospitals per inhabitant, and the birth rate is high, which matches some of the characteristics of the provinces with a high gender pay gap. On the other hand, foreign exchange earnings are above the third quartile, the highest in China. In addition to being one of the richest provinces in terms of GDP per capita, it has high electricity consumption per capita and a high unemployment rate compared with the other provinces.
Analyzing the Yangtze Economic Zone, we find provinces that have historically had a higher gender pay gap, such as Sichuan (Cluster 6), Chongqing, Hubei, and Anhui (Cluster 5), and Jiangsu (Cluster 3). Except for Sichuan and Chongqing, which showed a medium gender pay gap in 2018, the others (Hubei, Anhui, and Jiangsu) have continued to show an upward trend. They are the provinces with the highest gender pay gaps in China, with the exception of Fujian on the coast, which was not included in the CHIPS database. Note that, in general, the provinces belonging to Cluster 5 are below the average for every factor. In particular, Hubei and Anhui have a high gender pay gap and show below-average foreign exchange earnings from international tourism, have gas consumption and hospitals per million inhabitants below the first quartile, and population and GDP above the third quartile. In addition, Sichuan, in Cluster 6, is a large province in terms of population and GDP, but with very low energy consumption and low foreign exchange earnings from international tourism. Jiangsu, in Cluster 3, is also a large province with a high GDP and more than 80 million inhabitants, a birth rate under the first quartile, hospital numbers below the average, average dependency on gas but high electricity consumption, and below-average foreign exchange earnings from international tourism.
Jiangxi, in the Yangtze River area, belongs to Cluster 5 and is another province in this cluster with a high gender pay gap. It is one of the provinces with a population around the average for China, with electricity consumption per capita below the first quartile and gas consumption per capita almost at the minimum. Foreign exchange earnings from international tourism are low, in the first quartile, as is the number of hospitals, which is below the first quartile. The birth rate in Jiangxi is one of the highest in the country.
The other two provinces with significant differences in wages were Shandong and Hebei, both in Cluster 3. Shandong has more than 100 million inhabitants and Hebei more than 75 million. Gas consumption is low, the number of hospitals is below the average, and foreign exchange earnings from international tourism are not high (below the median in Shandong and below the first quartile in Hebei).
Moreover, Henan (Cluster 6) suffered from a high gender pay gap in 2018, although during the previous surveys it was not very high compared with other provinces. It is the third-largest province in terms of population, with more than 96 million inhabitants, and a GDP above the third quartile. Its gas consumption is below the median, hospitals per million inhabitants are below the first quartile, and in the second quartile it has the lowest foreign exchange earnings from international tourism.
Finally, two provinces showed large differences in wages between genders until 2013, but followed different trends in the last survey. On the one hand, in Guangdong (Cluster 7) in the South the gender pay gap has narrowed to some extent, while, on the other hand, it has become wider in Shanxi (Cluster 4) in the North. As in other provinces with a high gender pay gap, foreign exchange earnings from international tourism in Shanxi in 2018 were below the first quartile. Meanwhile, tourism has become an important source of foreign exchange in Guangdong, the third province in China after Beijing and Shanghai. It is the most populous province in China, with the highest GDP; however, gas consumption and number of hospitals are low, and the birth rate is high.
Note that the analysis carried out did not attempt to establish any causal relationship between the gender pay gap and the variables and factors considered. However, in general, all these provinces with a high gender pay gap, except for the exceptions mentioned, have a lower level of foreign investment, and are among those with lower energy consumption per capita (especially gas) and lower social development (especially as far as healthcare is concerned).
Discussion and Conclusions
Attention to gender equality is an important issue in the UN’s 2030 Sustainable Development Goals. With less than ten years remaining before the 2030 deadline, the study of the historical evolution of the gender pay gap from a spatial perspective and more recent socioeconomic development in China is particularly relevant. With data for 1995, 2002, and 2013 (CHIP, 2019) and the most up-to-date available data (from 2018; CFPS, 2020), this spatiotemporal multidimensional study on the gender pay gap in China shows a general upward trend. In global terms, the gender pay gap for annual earnings in these three years stands at 17.2%, 19.1%, and 23.2%, respectively, with the gap for hourly earnings standing at 15.8%, 17.3%, and 21.9%. In addition to these results, in 2018, the gender pay gap for China's annual earnings has reached 26.5% and for hourly wages, 20.9%. The gap is now greater than that in the European Union and the United States, whereas it was smaller than both in 1995. This upward trend in China’s gender pay gap from 1995 to 2018 is particularly worrying.
Reflecting rising wages, China's economy has developed rapidly since the beginning of the twenth-first century. This is due to reforms and a more open economic policy from the 1980s onwards, which has attracted more funds and involvement from international markets. However, these changes have brought their own challenges. With the transformation of China from a planned to a market economy, the state has less power to intervene in the market. This lack of control may have contributed to the widening of the gender pay gap along with the unequal socioeconomic development experienced by China's provinces.
The 1995–2018 spatiotemporal analysis shows that China's gender pay gap differs greatly for each of the provinces analyzed. This is particularly acute in the differences seen between the southern coastal and the northern interior provinces, and the differences seen between zones of highest and least economic development. The gap between the South and North is mainly reflected in the gender pay gap in hourly earnings. From 1995 to 2018, the gap in most northern provinces gradually decreased, while it increased in all southern provinces. In the less economically developed areas, it has remained at a constant level, while the gender pay gap between the more economically developed areas and the developed provinces (Beijing, Guangdong) has widened by varying degrees. From the perspective of the economic region, the gender pay gap in the Yangtze River Economic Zone is relatively wide, whereas provinces without relevant policies or geographic advantages have a smaller gap. This is a symptom of the lack of specific steps taken in these economic development plans to avoid the gender pay gap.
Trying to get a deeper understanding of why these differences in the gender pay gap of different areas of China exist, added to this classic spatial approach between southern coastal provinces and northern interior ones, and between zones of highest and lowest economic development, this article provides a novel grouping of Chinese provinces based on their socioeconomic characteristics. The five factors—wealth, size, energy consumption and transport, society, and unemployment—that summarize the 14 variables considered lead us to identify nine groups of provinces with similar characteristics. The socioeconomic clustering of China’s provinces developed in this study and its relationship with the gender pay gap allow us to conclude that this gap is moderately influenced by the conditions of regional economic development. In general terms, the gender pay gap increases with the size of the province in terms of GDP and population, noting that all provinces with more than 50 million inhabitants or with a production above 3,800,000 million yuan have a gender pay gap above the average. Similarly, provinces with birth rates below the first quartile presented a low gender pay gap. By contrast, we find that the higher the foreign exchange earnings from international tourism, the lower the gap, and that provinces with high gas consumption present a medium-low gender pay gap. The society factor is characterized by the number of hospitals per million inhabitants, identifying the fact that provinces with a high score have a low gender pay gap. Again, as stated earlier, it is important to emphasize that the linear correlation coefficient does not imply causality. Beijing and Shanghai (Cluster 1) have low gender differences in hourly wages, as do the northeastern provinces (Cluster 2), while Hubei and Anhui (Cluster 5) and Fujian (Cluster 6) have the highest.
The quite different spatial patterns and female-male ratios observed in the most recent article published by Bai, Lee, Liao, Wu, Xie and Zhou (2022) are due to methodological issues: their sample is based on online job seekers, resulting in a very low mean age (27 years for women and 29 years for men), and the working population as a whole is not well represented; the most recent annual salary is used as the person’s true salary, which, in itself, could already carry implicit differences in gender behavior. However, the conclusions of this current study may be limited by the small sample size observed in some provinces in the 2018 database. Moreover, the use of a median-based estimator of the gender pay gap instead of the most commonly used mean-based estimator could have excluded the influence of extreme values. Finally, this article does not include Moran’s I or a variogram estimation because the aim is not to see if the gender pay gap is spatially dependent or to study the structure of that dependency, but to see the relationship between the gender pay gap and the socioeconomic structure at the provincial level in China.
This article identifies many factors that affect the gender pay gap, but many others also exist. Spatial differences require different policies according to different development regions. This represents a challenge, as it calls for the formulation of relevant policies to reduce this gap. It is important to pay more attention to the balance of regional development, particularly in rapidly developing regions. In these regions, where industrial changes and staff turnover are rapid, companies are more likely to face discrimination issues when choosing employees to gain a competitive advantage. It is important to reward companies with moderate gender pay gaps and establish systems to penalize those institutions and companies with high gender pay gaps, and companies must be encouraged to provide free training to all employees to ensure a greater balance of knowledge and skills. The formulation of laws requiring equal pay for men and women should be considered. Guo, Chen, and Zeng (2021), at a regional level, show that fintech promotes women’s entrepreneurship and employment, thereby improving their labor participation and wages, which leads to a reduction in the gender wage gap. Detailed measures are needed to reduce the wage gap, thus achieving gender pay equality and moving closer to total gender equality without forgetting that Chinese society is still dominated to a significant extent by the traditional assumptions and expectations of what Evans (2021) characterized as the “patchy patriarchy.
Acknowledgments
The authors acknowledge the support of the SUPERA COVID19 FUND, a joint tender offered by CRUE(Conference of Rectors of Spanish Universities), CSIC (the Spanish National Research Council) and Banco Santander (Santander Bank), through the project ROCOGIS (The Faces of Covid. Gender and Socioeconomic Impacts).
References
- Anderberg, M. R., & Blashfield, R. G. (1973). Cluster analysis. Thousand Oaks, CA: Sage Publications.
- Bai, W., Lee, Y. L., Liao, J., Wu, L., Xie, M., and Zhou, T. (2022). The gender pay gap in China: Insights from a Discrimination Perspective. Retrieved June 19, 2022, from https://arxiv.org/abs/2206.09306v1, [physics.soc-ph].
- CFPS. (2020). China family panel studies. 985 Program of Peking University; Institute of Social Science Survey of Peking University. Retrieved January 3, 2022, from https://isss.pku.edu.cn/cfps/index.html/.
-
Chen, Z., Ge, Y., Lai, H., & Wan, C. (2013). Globalization and gender wage inequality in China. World Development, 44, 256–266.
[https://doi.org/10.1016/j.worlddev.2012.11.007]
-
Chi, W., & Li., B. (2014). Trends in China’s gender employment and pay gap: Estimating gender pay gaps with employment selection. Journal of Comparative Economics, 42(3), 708–725.
[https://doi.org/10.1016/j.jce.2013.06.008]
- CHIP. (2020). Chinese Household Income Project. China Institute for Income Distribution. Retrieved January 25, 2021, from http://www.ciidbnu.org/chip/index.asp?lang=EN
- European Commission. (2020). The gender pay gap situation in the EU. Retrieved February 3, 2021, from https://ec.europa.eu/info/policies/justice-and-funda-mental-rights/gender-equality/equal-pay/gender-pay-gap-situation-eu_en
-
Evans, H. (2021). “Patchy Patriarchy” and the Shifting Fortunes of the CCP’s Promise of Gender Equality since 1921. The China Quarterly, 248(S1), 95-115.
[https://doi.org/10.1017/S0305741021000709]
- Everitt, B. S. (1993). Cluster analysis (3rd ed.). London: Edward Arnold.
- Gorsuch, R. L. (1983). Factor analysis (2nd ed.). Hillsdale, NJ: Lawrence Erlbaum Associates.
-
Gorsuch, R. L. (1990). Common factor analysis versus component analysis: Some well and little known facts. Multivariate Behavioral Research, 25(1), 33–39.
[https://doi.org/10.1207/s15327906mbr2501_3]
-
Guo, Q., Chen, S., & Zeng, X. (2021). Does Fintech Narrow the Gender Wage Gap? Evidence from China. China & World Economy, 29(4), 142–166.
[https://doi.org/10.1111/cwe.12382]
-
Gustafsson, B., & Li, S. (2000). Economic transformation and the gender earnings gap in urban China. Journal of Population Economics, 13(2), 305–329.
[https://doi.org/10.1007/s001480050140]
- Hair, J. F., Anderson, R. E., Tatham, R. L., & Black, W. C. (1998). Multivariate data analysis. Hoboken, NJ: Prentice Hall.
-
Hare, D. (2019). Decomposing growth in the gender wage gap in urban China: 1989–2011. Economics of Transition and Institutional Change, 27(4), 915–941.
[https://doi.org/10.1111/ecot.12222]
- Harman, H. H. (1976). Modern factor analysis (3rd rev. ed.). Chicago, IL: The University of Chicago Press.
-
Hughes, J., & Maurer‐Fazio, M. (2002). Effects of marriage, education and occupation on the female/male wage gap in China. Pacific Economic Review, 7(1), 137–156.
[https://doi.org/10.1111/1468-0106.00156]
- Jianhua, Z., & Jun, G. (2019). Study on the problems and the countermeasures of the unbalanced development of regional economy in the 40 years of China's reform and opening up. Contemporary Economic Management, 41, 9–14. 张荐华, & 高军. (2019). 中国改革开放 40 年中的区域经济发展不平衡问题与对策研究. 当代经济管理, 41(2), 9-14.
-
Jollife, I. T. (1986). Principal component analysis. New York, NY: Springer.
[https://doi.org/10.1007/978-1-4757-1904-8]
- Ju, P. (2020). Analysis of the measurement of imbalanced regional economic development and its influencing factors in China. (Unpublished doctoral dissertation). Liao Ning University). In Chinese. 潘桔. (2020). 中国区域经济发展不平衡测度及影响因素分析 (Doctoral dissertation, 辽宁大学).
-
Kaiser, H. F. (1958). The varimax criterion for analytic rotation in factor analysis. Psychometrika, 23(3), 187–200.
[https://doi.org/10.1007/BF02289233]
-
Knight, J., & Song, L. (2003). Increasing urban wage inequality in China: Extent, elements and evaluation. Economics of Transition, 11(4), 597–619.
[https://doi.org/10.1111/j.0967-0750.2003.00168.x]
-
Larraz, B., & Pavia, J. M. (2010). Classifying regions for European development funding. European Urban and Regional Studies, 17(1), 99–106.
[https://doi.org/10.1177/0969776409350679]
-
Manly, B. F. (2004). Multivariate statistical methods: A primer (3rd ed.). London: Chapman and Hall/CRC.
[https://doi.org/10.1201/b16974]
-
McDonald, R. P., & Burr, E. J. (1967). A comparison of four methods of constructing factor scores. Psychometrika, 32(4), 381–401.
[https://doi.org/10.1007/BF02289653]
- National Population Census. (2010). Sixth National Population Census of China Database. National Bureau of Statistics. Retrieved July 17, 2021, from http://www.stats.gov.cn/english/Statisticaldata/CensusData/rkpc2010/indexch.htm/
- Naughton, B. (2007). The Chinese economy: Transitions and growth. Cambridge, MA: MIT Press.
-
Ng, Y. C. (2004). Economic development, human capital, and gender earnings differentials in China. Economics of Education Review, 23(6), 587–603.
[https://doi.org/10.1016/j.econedurev.2004.03.004]
-
Ng, Y. C. (2007). Gender earnings differentials and regional economic development in urban China, 1988–97. Review of Income and Wealth, 53(1), 148–166.
[https://doi.org/10.1111/j.1475-4991.2007.00221.x]
- OECD. (2020). Gender wage gap (indicator). Retrieved July 19, 2021, from https://doi.org/10.1787/7cee77aa-en/
- Romesburg, H. C. (2004). Cluster analysis for researchers. Morrisville, NC: LULU Press.
-
Stevens, J. P. (2001). Applied multivariate statistics for the social sciences. Hillsdale, NJ: Lawrence Erlbaum Associates.
[https://doi.org/10.4324/9781410604491]
- Song, Z. (2013). Economic open up, market segmentation and unbalanced regional economic growth in China (unpublished doctoral dissertation). Shan Dong University, Jinan, China. 宋志涛. (2012). 经济开放, 市场分割与我国地区经济发展不平衡 (Doctoral dissertation, 山东大学).
- UN. (2015). 2030 Agenda on sustainable development. Retrieved July 30, 2021, from https://www.un.org/sustainabledevelopment/es/2015/09/la-asamblea-general-adopta-la-agenda-2030-para-el-desarrollo-sostenible/
- UN. (2020). Women and girls – closing the gender gap. UN75, 2020 and beyond. Retrieved July 3, 2021, from https://www.un.org/sites/un2.un.org/files/2020/03/un75_gender.pdf
-
Whalley, J., & Xing, C. (2016). Privatización y desigualdad salarial en la China urbana. Revista Internacional del Trabajo, 135(1), 61–77. In Spanish.
[https://doi.org/10.1111/ilrs.12002]
- World Economic Forum. (2020). Global gender gap report 2020. Geneva, Switzerland: Edit World Economic Forum.
-
Xiu, L., & Gunderson, M. (2013). Gender earnings differences in China: Base pay, performance pay, and total pay. Contemporary Economic Policy, 31(1), 235–254.
[https://doi.org/10.1111/j.1465-7287.2011.00307.x]
Appendix
Supplementary material

Variables used for clustering the provinces of China in 2018Note. The data of 2018 are revised according to the data of the fourth national economic census.

Correlation coefficients between gender pay gaps on annual and hourly wages and factors and variables, respectively
Biographical Note: Beatriz Larraz has a degree in Mathematical Sciences from the Universidad Autónoma de Madrid and a PhD in Economic and Business Sciences from the Universidad de Castilla-La Mancha. She is currently Professor of Statistics at the Toledo Faculty of Legal and Social Sciences, at the Universidad de Castilla-La Mancha (Spain). Among her main lines of research are spatial statistics, inequality measures, gender equality, real estate market, environmental economics and electoral processes. She has published thirteen books, ten book chapters and more than forty articles in internationally recognized journals.
Biographical Note: Sun Xinbo has a Master’s degree in Business Strategy and Marketing and a Bachelor’s degree in business administration from the University of Castilla La Mancha in Spain. He is now a PhD student in Economics. He has internship experience in private companies and is engaged in Internet e-commerce, company data analysis and company strategic planning.